Meredith Martin is an associate professor of English and serves as the Faculty Director for The Center for Digital Humanities (CDH), which she founded in 2014. She is also the inaugural Faculty Director of the first Graduate Certificate in Digital Humanities at Princeton and serves as an advisor for undergraduate students pursuing Certificates such as Applications in Computer Science, Statistics in Machine Learning, Journalism, or Technology and Society.
The CDH at Princeton is a research center that approaches various digital technologies from a humanistic perspective. Its role is to develop better practices in academia, technological development, and research. The CDH currently runs research projects, consultations, undergraduate and graduate-level courses, and events that educate the Princeton community about the digital humanities.
The Daily Princetonian sat down with Martin to discuss the importance of the digital humanities and the impact of the CDH at Princeton. This conversation has been edited for clarity and concision.
The Daily Princetonian: What does the term “digital humanities” encompass?
Meredith Martin: At Princeton, we have a really specific definition of digital humanities. I think any institution has to define digital humanities depending on the landscape of the research computing, the university library, and whether or not their university has a virtual reality, teaching, or learning lab. So, digital humanities necessarily becomes part of the strategic framework and looks like the institution. Traditionally, digital humanities departments grew out of university libraries as parts of library centers — like digital scholarly services — and ours did not. Ours grew out of a collaboration of faculty who worked in the humanities, social sciences, and computer science with staff from the library and the Office of Information Technology, all of whom wanted to use computational tools to accelerate research across many domains. We hoped to build collaborative environments where we could think through data-driven approaches to the human record — be that in sociology, social sciences, or humanities — in order to think through a more just future.
Our idea is not that humanists and social scientists are just using these tools that computer scientists are building for us, but that we’re actually learning enough about the tools, and enough about the methods and algorithms and all the approaches, to critique them. We want to bring a human lens and argue for humans during the process of technological development. Also, we’re co-creating. We’re working in collaboration with research software engineers and computer scientists. We’re teaching Princeton undergrads and Princeton graduate students how working collaboratively and interdisciplinarily — kind of “transdisciplinary” — across units and divisions is the way forward.
DP: Why do you think it’s important to learn about technology, and more specifically data science, through a humanistic perspective?
MM: Well, I think a humanistic approach to data science will help people understand the power structures that underpin all of the mechanisms that already underpin their daily lives. It’s important to have an approach to data that is not taking it as a given, but as an understanding that all data comes from somewhere and is a proxy for power structures. Those are fundamental lessons that you have to understand if you’re going to work in these fields. And so, a humanistic approach is not that different from any other very responsible approach to technology. You want to be able to understand the source material, you want to have context for the data, and you want to be able to close-read it. Is the dataset complete? Incomplete? Is it nefarious? Does it have a political reason why it’s showing up in data and statistical services and where does it come from?
You want to do the source criticism of your dataset before you perform something on it. Just apply critical thinking: the things that humanities is known for. Mine are all C’s because it helps me remember them: close reading, context, critical thinking, and creatively and effectively communicating what your findings are. It’s not just something where, for instance, you’re using a data-driven technology to build a tool that’s useful for some people. We should actually have transparency about how those things are being used — how people’s data is being used, how it’s extracted, and how it's communicated. It’s generally not something that people think about when they’re in an applied math class, because math has truth. Data is not math. Data is kind of in the middle. And so, I think we’re in that ambivalent middle, where we can help people understand how to ask questions about their data and how to ask questions about the reasons behind the resulting technologies that they might build with the resulting algorithms. But I think we’re also really excited about the ways that technology can help people understand the human record in really interesting and new, revolutionary ways.
DP: Is there anything you would want to change about the way that AI and machine learning are currently being taught at Princeton or other universities in general?
MM: I think we need to do a lot more of it at Princeton. I think we need to teach it in an integrated way across departments in a program where we’re able to work in an interdisciplinary way. If we had a minor that allowed people to think through and critically apply machine learning to their majors, that would be fantastic. My recommendation would be to hire a lot more people because at the end of the day, this is a big research area, and we can’t really expect our current professors to do this and all the other things they already do really well. I think if we could hire many more people to help equip the undergrads and the graduate students, but also help equip our current faculty to be prepared for what is coming, that would be really helpful. And when I say for what’s coming, I mean a generation of students coming in five or six years time who will have had — for better or worse — generative AI integrated into some aspects of their secondary school or even earlier education. We have to be at least as media-literate as our students, and that’s going to take an investment.
There’s nowhere like Princeton, so I don’t want to be like any other school, but there are undergraduate programs or interdisciplinary centers that I think are really useful models that we might be able to emulate. But I think we’re going to have to do whatever we have to do in a very Princeton-specific way.
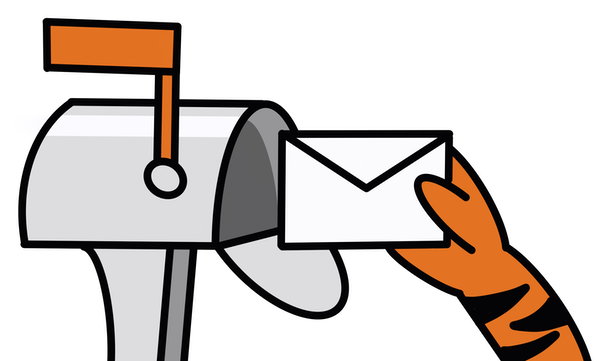
DP: What would you say is the role that the Center for Digital Humanities is playing in modern technology, especially now with all of these new AI models being developed?
MM: November 2022 was when we first saw ChatGPT. But even before that, there were lots of scholars who were working on large language models and thinking about stochastic parrots and the dangers of what used to be called big data. They also considered the possibilities and opportunities of applying machine learning technologies, which are often now branded as artificial intelligence, to larger than normal datasets. Humanities and social science professors and researchers have been using data-driven technologies and computational approaches for decades. The acceleration of transformer technology that sort of leapt over into what people are now calling artificial intelligence certainly made bigger and faster things possible. But it’s not necessarily a different angle than the one that we started out having, which is that humanists can understand cultural materials as data in a better, more nuanced way than computer scientists can.
For people who are working in natural language processing, the fields of computational linguistics, and our thinking about what these large language models can do, we’ve always felt like empowering humanities scholars to be in that room. Say, for art history, if you’re working in computer vision and you want to look at a whole bunch of paintings and come up with some theory, it probably would be good for you to talk to the expert in those paintings because they’re right there. So our whole job is not necessarily to teach people how to use the technology, but to try to build these bridges across a variety of disciplines. That way, our computer scientists are better and more prepared and our humanists feel empowered to be part of those conversations.
DP: What are some future political or ethical considerations society should have when developing new technology?
MM: I think that there are a lot of things happening very quickly and that there are also a lot of people who are benefiting very quickly. And then there are a lot of people who have already been harmed by these technologies and who continue to be harmed by the technology. So the tension is to kind of strike a middle path. And I think humanists in general, like I said before, we’re very used to living in that ambivalent middle. We’re used to ambiguity. We’re used to things not fitting very well into a spreadsheet and we understand what kinds of interpretive choices we have to make. So I think, in general, we are absolutely against technology that causes harm. We tend, as a center, to want to work with collaborators who are thinking about the benefits of the technology for a broader audience, even more than just for their research. So the software that we write and the things that we try to build with faculty tend to be replicable for other faculty down the road. You know, I think sharing, transparency, and communicating what we’re doing and why we’re doing it is part of what we do.
That said, the companies that are benefiting right now from this recent AI boom are not doing the best job. In fact, they’re benefiting from the fact that the hype around this particular moment has been describing what’s been happening as a black box — that it’s really difficult to understand. Actually, it’s not very difficult to understand. And this sort of shiny-eyed excitement over it is a hype that takes away from the excitement of what’s actually happening.
We’re not reaching out and creating literacy around what those processes look like and where and how they’re impacting the environment and workers. So I think a commitment to basic data literacy is as important as close reading, critical thinking, and writing, which are all the regular things that a humanities department would do.
DP: What would you say to students who are interested in studying this mix between the humanities and technologies such as AI?
MM: I think that they should absolutely look at the course list that the Center for Digital Humanities curates every term. We don’t have a minor, but we try to be really selective in the courses that we think would be interesting to the students that we see in our Introduction to Digital Humanities course. We have a graduate certificate now and it’s CDH Computational and Data Humanities.
Even if you can’t take all the courses that you want and even if it takes us a couple more years to build that curriculum, we have an amazing group of speakers that come to campus. There are also many courses through the library and through PICSciE for research computing. And if there’s really a specific thing that you want to do in a class, book a consultation at the CDH and say, “I want to do this cool project.” We work with a lot of undergrads on their independent work projects, especially if they already have a little bit of an interest in quantitative approaches.
Judy Gao is a staff Features writer for the 'Prince.'
Please send any corrections to corrections[at]dailyprincetonian.com.