On Wednesday, Nov. 16, Dr. Uyi Stewart, Chief Data and Technology Officer at Data.org, delivered a lecture in Robertson Hall titled “Data Science: The New Frontier in Global Health and Development,” that revolved around the potential of data science to address a sprawling range of contemporary global issues, including infectious diseases, economic vulnerabilities, drug treatments, and healthcare access, among other pressing areas of importance.
Data.org is a nonprofit organization that strives to “democratize and reimagine the use of data to tackle society’s greatest challenges and improve lives across the globe,” according to their website.
Stewart previously served as Director of Strategy, Data and Analytics in Global Development at the Bill and Melinda Gates Foundation and co-founded IBM Research – Africa, serving as the platform’s chief scientist and capitalizing on big data to help reign in the Ebola outbreak that erupted in West Africa in 2014.
The Daily Princetonian sat down with Stewart to discuss his past work and how data science can be used to address urgent global humanitarian issues. This interview has been edited for clarity and concision.
The Daily Princetonian: As the chief data and technology officer at Data.org, what does your nonprofit organization aim to accomplish?
Dr. Uyi Stewart: In the social impact space, there’s a lot of fragmentation. So social impact organizations are the folks using data and other means to address inequities around the world. But what you find is that everyone is doing their own thing, so there’s a lot of fragmentation. And while it has intended to do good, good has not been achieved. It’s in small bits. What is needed is a way to combine all of these efforts while remaining true to objectives but finding a way to maintain economies of scale. That’s the problem and the ambition. We are a platform to coordinate the field to drive partnerships towards data for social impact. That’s really our goal. And in the process, to train one million purpose-driven data scientists in 10 years.
DP: You played a pivotal role in pioneering the use of vast data to combat the Ebola outbreak in West Africa during your stint as the chief scientist of IBM Research – Africa, which you also cofounded. What was that experience like and what were some of the main challenges that you encountered?
US: That was one of the most challenging things that I’ve had to go through as a data scientist. Today, it’s very common to see the use of data to model the infection rate or the trajectory of the spread of the disease, as we’ve seen from [COVID-19]. So you look at infection rates, infection patterns, spikes, and ebbs. But in 2014, when Ebola struck in West Africa, the use of big data to model disease was very nascent. It was a feat of epidemiology and even getting around to do that was challenging at the time. There was access to data, to answer your question, but the biggest challenge was the stigma that is associated with Ebola.
The prevailing culture in West Africa is such that burial practices require a rite of passage. When relatives die, there is a rite of passage and that passage involves washing of the clothes. But Ebola is a disease that spreads through touch. If you touch an infected person, you’re going to contract Ebola. So it was a clash between a disease that spreads by contact and the traditional habits of the people. The big challenge, therefore, was how do you use innovation technology to affect behavior change? That was the big issue. And that was the work that we were able to successfully do in Sierra Leone.
DP: What would you consider to be some of the most pressing issues facing humanity today that you believe data science is uniquely positioned to address?
US: A lot ... I can talk about gender inequality. Not just in the [United States], but throughout the world. In India and across countries in Africa, there’s just massive gender inequality — especially with education and even in healthcare — that is staring us in the face.
But there’s climate. In fact, the climate crisis is a health crisis. And I think we are not stepping up to the plate. There is inequity in the way knowledge is disseminated around the world. The world wide web is English-dominated and therefore billions of people are being left out of this information corridor. I can go on and on and on, but these are some of the challenges we face around the world right now.
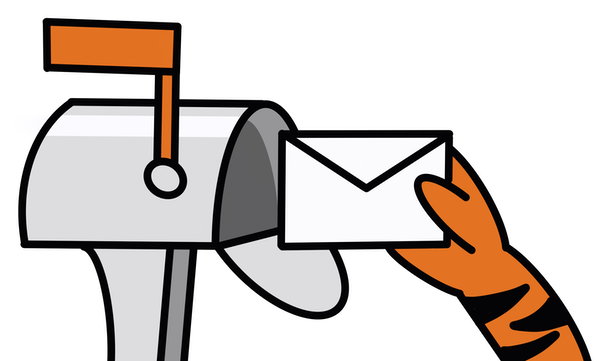
DP: Yes, those seem to be very extensive challenges that represent a global phenomenon, not just issues confined to a particular area or place.
US: That’s right. I isolated these because you asked where data can help. So I think data can help to address the inequities in gender, inequities in health, and the inequities we see in language, in order to build better translation models.
DP: Could you expound on some of the novel data science techniques and technologies that have emerged recently and how they could be deployed with respect to identifying mechanisms of disease transmission, catalyzing drug development, and improving access to health resources, among other applications?
US: I can talk about the ability to unlock new datasets, which is really phenomenal. One of the things that’s happened in the last maybe, five to 10 years, is to take a dataset — like health information — and overlay on that what we call geospatial data, data about movement. What has been done is that now we can begin to identify specific pockets, or what we call heat maps. Before, you could do a blanket treatment of a problem. So say, for example, there is dengue fever in an entire area. But the thing is that one size doesn’t fit all because dengue fever may be intense in a specific area. But previously, data did not exist around geospatial movement. All you had was data on dengue fever. But now you have the social determinants of health data, but you also have movement data, coming from cell towers and cell phones.
When you overlay geospatial data, you can begin to get a degree of precision in your health intervention. Rather than a blanket treatment, you can now be more specific — what we call targeted intervention. That’s an innovation that has just happened recently. I think that’s great to help us to achieve better health outcomes, through targeted interventions, as an example.
DP: Lastly, in a data-saturated world, do you think that innovative data science techniques are sufficient to tackle the superabundance of data? What challenges remain to be overcome?
US: We are still on the cusp of causal inference. A lot of the analyses we see today are all looking for correlations in datasets by looking for interpretive features within the dataset. The next big thing we’re looking for, at least in the social impact sector, is what is a causal inference? If I see X and Y, can I conclude the trigger from the data?
For example, boys walk five miles to school — I’m just making this up — and girls walk seven miles to school. At the end of the year, we see results as test scores come back: boys are doing better, girls are doing worse. The question is, how do we explain these findings? Is there a causal relationship, can you conclude that those extra two miles is a reason that girls are performing poorer in test scores? Right now, data science cannot give you with a degree of precision the exact conclusion yet. It is about probability; we can tell you that in all likelihood, it’s because of the two miles. But we cannot say it with certainty. So we are still figuring out how to be much more prescriptive from the data in terms of causal inference, as an example.
Implicit in what I just explained is this big issue of how do you go from insights into action. If I find out that, ‘Wow, because girls walked two extra miles, that has an impact on their ability to be successful at school,’ then what is the recommendation in terms of action? What do we do about it? This is called ‘impact’ — how do we mitigate that? Analysis is great, but it’s not sufficient. For us to complete the arc and really say data is really making a difference, we have to go the next mile of turning insights into action. And right now, it’s more of an art than a science.
Amy Ciceu is a senior writer who often covers research and COVID-19-related developments.
Please direct any corrections requests to corrections at dailyprincetonian.com.