With the window for redrawing voting districts upon us, the topic of gerrymandering should be on everyone’s radar. This article is the second in a series of three that will explore the need for structural reform in the electoral system, the power of data to achieve these ends, and the ongoing efforts at Princeton in the Electoral Innovation Lab. You can find the first article of the series here.
When we consider the role of data in American politics, it is beneficial to recognize what data is, and, as importantly, what it is not. Given that statistics by themselves are one dimensional, without adequate context data itself does not capture the deep complexities and subjective forces of the systems they quantitatively explore. In other words, data alone does not inherently represent objectivity.
A clear case in point lies in the treatment of data in the criminal justice system, where it is not only incapable of conveying complete stories, but it can also be a normalized source of bias and oppression.
This oppressive role of data began to really take hold following the 1890 census, as the racial demographics of the carceral system were debuted for the country to shallowly disseminate. For the first time, Black criminality appeared rooted in numbers: the Black body objectively occupied the most space in American prisons. Without any contextualization to this statistic, soundbites like this were not only used to reinforce and justify the incrimination of Black people, but Black culture as well.
We still see this pathological rhetoric continuing today. Statistics like “Black men are six times more likely to be incarcerated than white men” constantly justify racial inequities in the criminal justice system. When only tasked with confronting numbers alone, the preponderance of incarcerated Black bodies dangerously pins responsibility on the Black community itself.
Nevertheless, numbers do not spawn in a vacuum. When we fail to contextualize data in the criminal justice system, we expunge the targeted spotlight on Black communities that naturally reveals more crime. We ignore the disparate conditions that systemically reproduce crime, as data is often not as independent as it is represented to be. Simply put, the quasi-objectivity of data conveniently erases crucial nuance.
The quest then becomes to subjectify the objective. Therein exists an urgency for pioneers committed to justice to stake claim in data science and be the vanguard of much needed change. And as we await the release of the 2020 census results, we have the opportunity to do so in another crucial arena: the electoral system.
It is not uncommon to hear proverbial statements regarding voter turnout such as: younger voters do not show up to vote, white voters turn out at higher rates than any other demographic, and certain demographics do not cherish their patriotic duties like their older, wiser (and quite frankly whiter) counterparts.
Instead of buying into these claims, what we ought to consider instead are the structural bugs in the electoral system that result in voter suppression. Take, for example, the recent voting laws passed in the Georgia legislature. Or consider the rampant gerrymandering that draws voting districts to strategically marginalize targeted communities from being heard.
In this timely year for electoral reform, it is important that we do not allow for these statistical soundbites to be further normalized. We must complicate them by intentionally contextualizing the structures from which they emanate. It is at this important juncture of the upcoming release of the 2020 census and the subsequent redistricting process that we have the option to probe deeper and distill what the data truly signifies, rather than taking it at face value.
One organization on campus that has been doing this work is the Electoral Innovation Lab, which analyzes data in a just, nonpartisan manner to find flaws in the electoral system. When numbers are taken into account in this conscious way, they can be translated into laws that reflect that. I spoke with Amanda Kmetz, a data engineer at the Princeton Gerrymandering Project, to understand the prospects for meaningful change through this progressive practice.
Given that much of the data that the Lab uses comes from the U.S. Census Bureau, the first way they contextualize the numbers is by recognizing their limitations. Oftentimes people are undercounted or overcounted, skewing the accuracy of the very numbers used to redistrict. Moreover, the rather arbitrary constructions of race lead to definitions that vary across districts. Kmetz noted that while Black may be categorized as “all people who identify as Black or African American, regardless of ethnicity” in one district, another may use the metric of “people who identify as Black or African American alone (not in combination with any other race), but not Hispanic or Latino.”
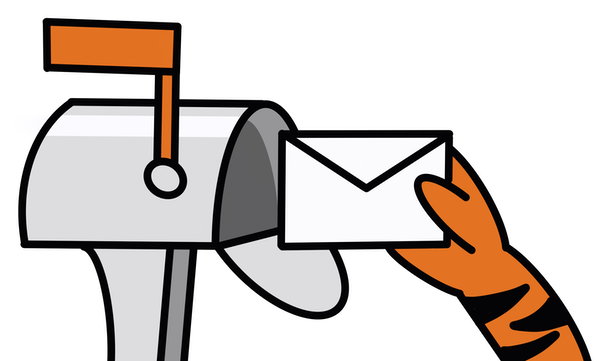
Even before using data in their work, the team at the Lab pays close attention to who the numbers represent and the voices they may inevitably erase. And as they seek to make use of data thereafter, that same prudence informs their work, as their data science team works hand in hand with the legal and research arms at the Lab.
For example, Kmetz outlined a problem in the electoral system that affects communities nationwide: there is currently not a centralized database for election results on the precinct level. As a result, the impact that smaller communities have in elections is obscured. Kmetz stated that this “makes it difficult to determine how changing district lines could impact elections”.
So, rather than taking very zoomed-out election results as they are, the Lab started a project called OpenPrecincts. Working with local organizations to ensure their use of data is collaborative, transparent, and just, OpenPrecincts is a database that offers precinct-level election results for all 50 states. That way, context can be attributed to data, which can help explain disparities in voter turnout, the prevalence of gerrymandering, and other areas for structural change.
Initiatives like these are key to a nonpartisan and just understanding of the political landscape around us. It is not enough to take data points as unequivocal truths without questioning the structures that create them. The progress of marginalized communities, from criminal justice reform to electoral reform, rests on being able to achieve this level of contextualization.
Gerrymandering and redistricting will fill media sites with data this spring and summer, often without the needed context, as media outlets take advantage of presenting complex topics to general audiences. As redistricting rolls around, I urge everyone to consider the so-called objective facts that you may hear and think about what they truly reveal about current systems and, of course, prospects for change.
Collin Riggins is a first-year student from Kansas City, Mo. He can be reached at riggins@princeton.edu.
Jason Rhode, the National Coordinator, and Amanda Kmetz, a Data Engineer at the Princeton Gerrymandering Project, provided guidance in writing this piece. They can be reached at jrhode@princeton.edu and akmetz@princeton.edu respectively.