Neuroscience professor Samuel S. Wang uses his mathematical skills and legal passions to help ensure voters choose their politicians, not the other way around.
His team’s paper, “An Antidote for Gobbledygook: Organizing the Judge’s Partisan Gerrymandering Toolkit into a Two-Part Framework,” won a top prize at a gerrymandering competition last week.
The paper was part of the Princeton Gerrymandering Project, which analyzes individual states’ courts, polling data, and legislative data from every state, Washington D.C., and Puerto Rico.
Through the project’s work, Wang’s team strove to craft a legal theory that can be used by advocates across the United States to target its most gerrymandered districts.
“I got very interested in applying math skills to try to fix what I thought was a problem in U.S. democracy,” said Wang, who founded the Princeton Gerrymandering Project in 2016.
“We were trying to take extreme math knowledge and repackage it in a way that didn’t lose math rigors but [is] more palatable to a judge,” said Ben Williams, a legal analyst who worked on the paper with Wang.
“Gerrymandering takes the ways that people live and vote and uses that information against people,” Williams said. He added that the gerrymanderer “weaponizes” it to enhance the power of the ruling party, with lasting implications.
Williams said he’s inspired to fight for accurate democratic representation and the ability for people to exercise their voices at the ballot boxes.
Suzanne Almeida, Redistricting and Representation Counsel at Common Cause, the organization that sponsored the research competition, praised the paper as a “Swiss Army knife” paper that combines different academic approaches to solve a complex national problem.
There were 11 entries to the contest and five finalists. Judges for the contest included UC Irvine Law School Dean Erwin Chemerinsky, Office of Congressional Ethics Board of Directors member Allison Hayward, Brennan Center for Justice Senior Counsel Michael Li, and Tufts University associate professor of mathematics Moon Duchin.
Brian Remlinger, who worked with Wang and Williams on the paper, was not available for comment at the time of publication.
Wang hopes to release the data project by the end of 2020.
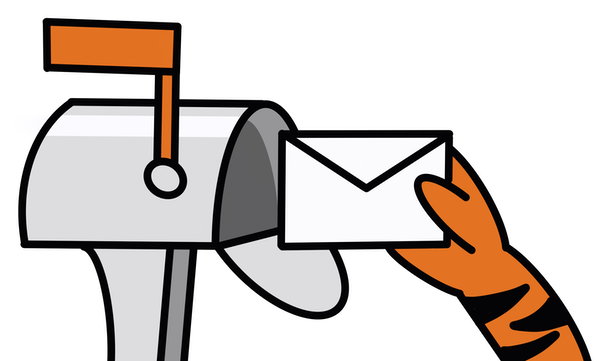